Planting a SEED of Vision in Large Language Model
For any inquiries, please email seed-x@googlegroups.com
Preface
We present SEED, an elaborate image tokenizer that empowers Large Language Models (LLMs) with the emergent ability to SEE and Draw at the same time. Research on image tokenizers has previously reached an impasse, as frameworks employing quantized visual tokens have lost prominence due to subpar performance and convergence in multimodal comprehension (compared to BLIP-2, etc.) or generation (compared to Stable Diffusion, etc.). Despite the limitations, we remain confident in its natural capacity to unify visual and textual representations, facilitating scalable multimodal training with LLM's original recipe.
SEED Tokenizer
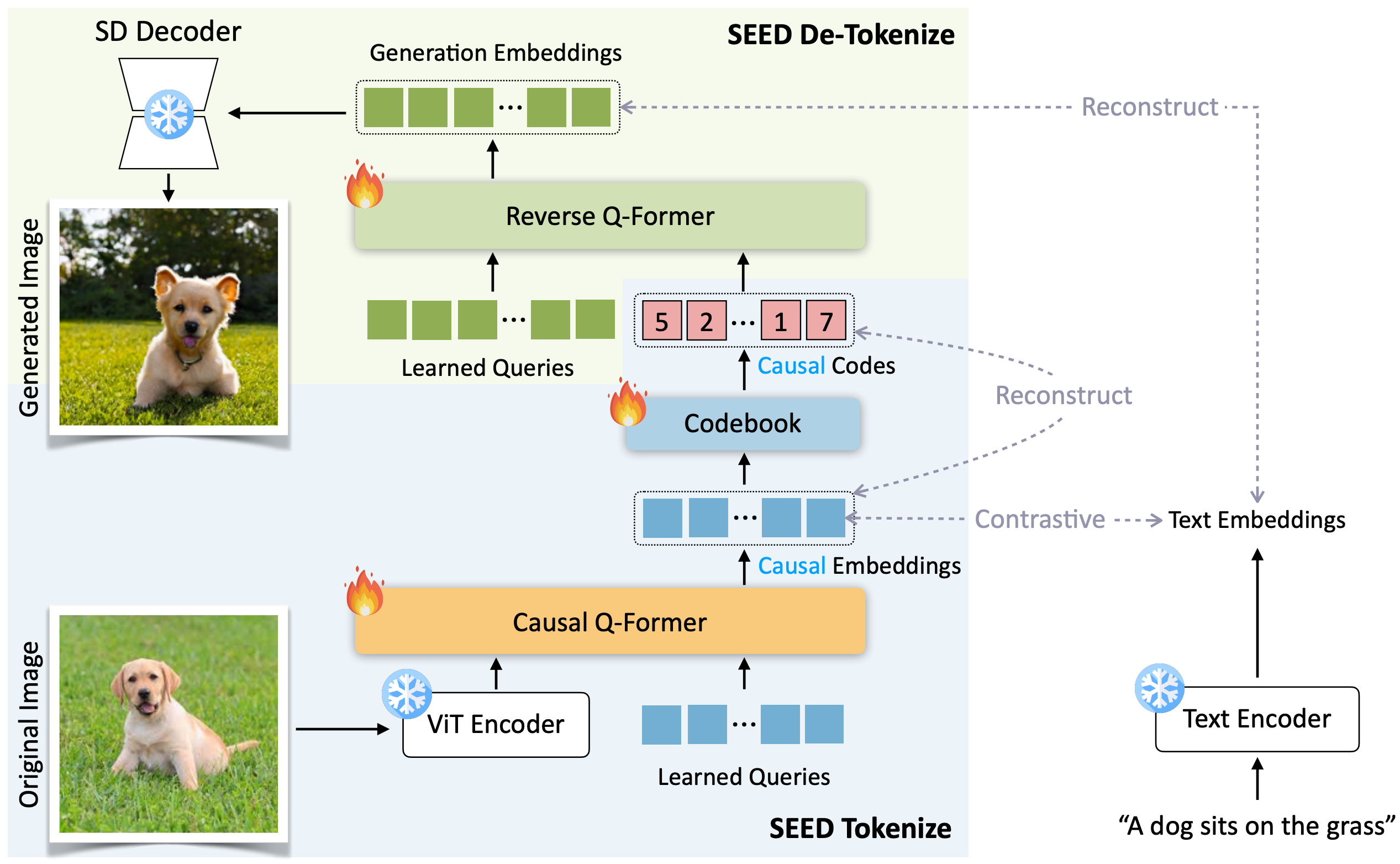
We identify two crucial principles for the architecture and training of SEED that effectively ease subsequent alignment with LLMs.
- Causal Q-Former: Image tokens should be independent of 2D physical patch positions and instead be produced with a 1D causal dependency, exhibiting intrinsic interdependence that aligns with the left-to-right autoregressive prediction mechanism in LLMs.
- Contrastive and reconstruction learning targets: Image tokens should capture high-level semantics consistent with the degree of semantic abstraction in words, and be optimized for both discriminativeness and reconstruction during the tokenizer training phase.
The working mechanism of SEED:
- Tokenize: Causal Q-Former converts 2D raster-ordered features produced by the ViT encoder into a sequence of causal semantic embeddings, which are further discretized by the VQ Codebook.
- De-Tokenize: The discrete visual codes are decoded into generation embeddings via Reverse Q-Former. The generation embeddings are aligned with the latent space of SD so that realistic images with consistent semantics can be generated using the off-the-shelf SD-UNet.
Evaluate the discriminativeness of SEED tokens

Evaluate the reconstruction ability of SEED tokens
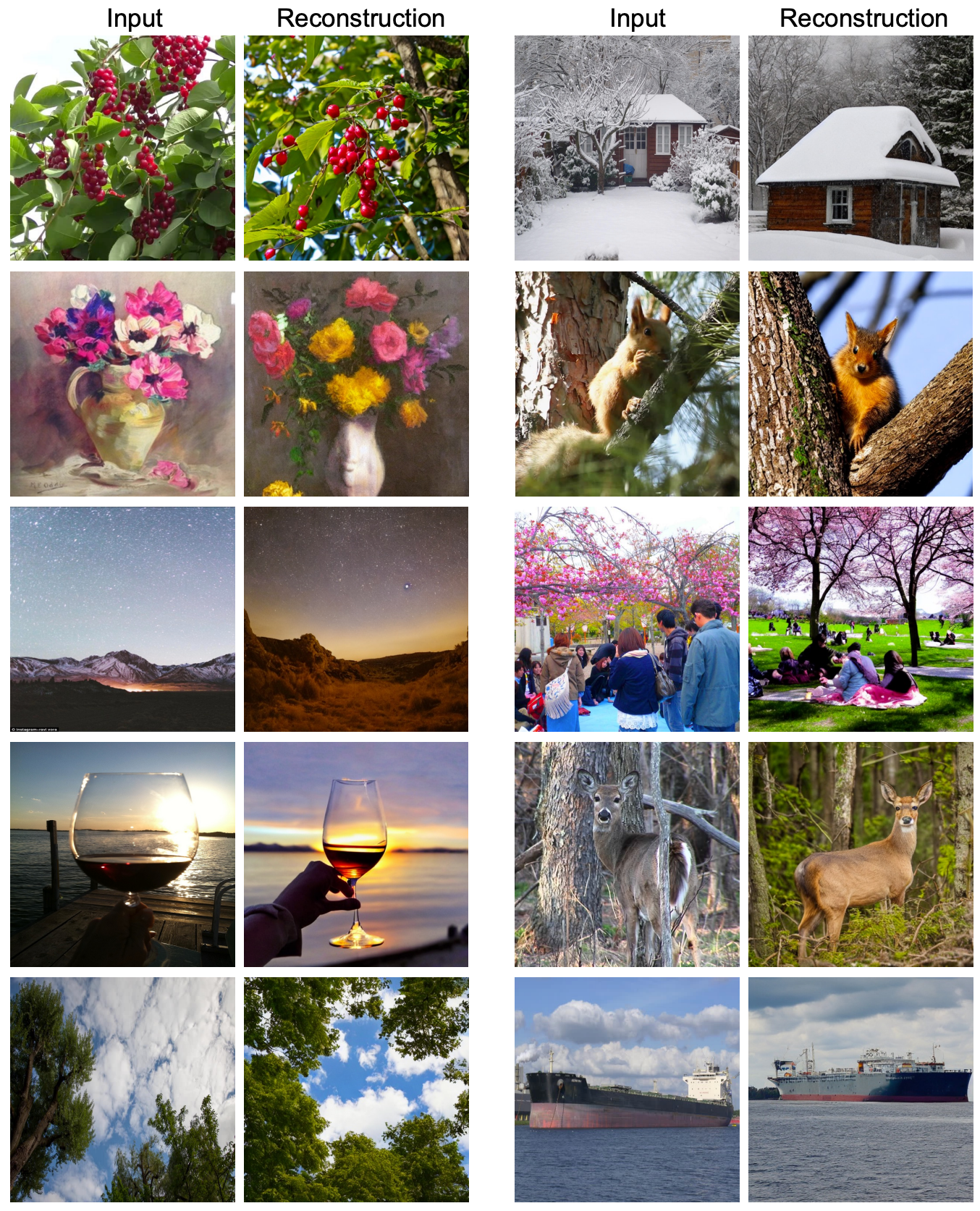
SEED for LLM
As a result, the off-the-shelf LLM is able to perform both image-to-text and text-to-image generation by incorporating our SEED through efficient LoRA tuning. We present SEED-OPT2.7B, which was trained in only 44 hours using 64 V100 GPUs and 5M image-caption pairs.
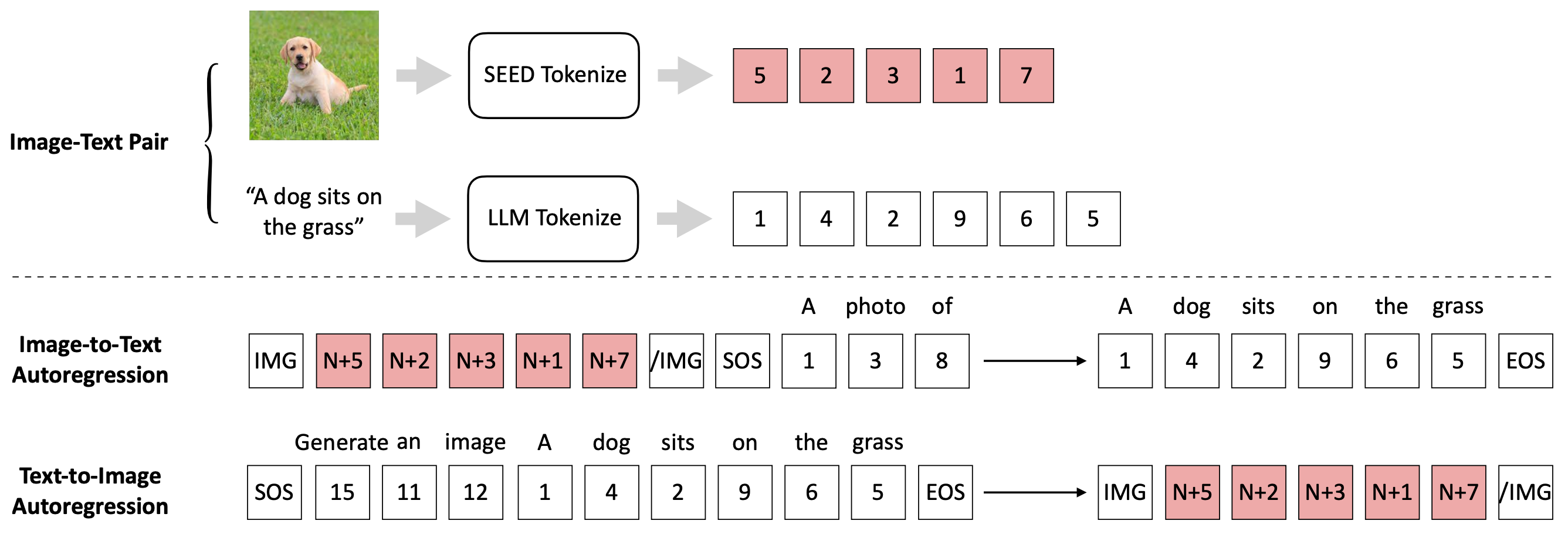
The trained SEED-OPT2.7B is capable of both image captioning (image-to-text) and image generation (text-to-image). More importantly, the model is able to perform open-ended Visual Question Answering (image&text-to-text), which can be considered an emergent ability as we did not use any VQA dataset for training. See the results below.
Qualitative evaluation: image comprehension
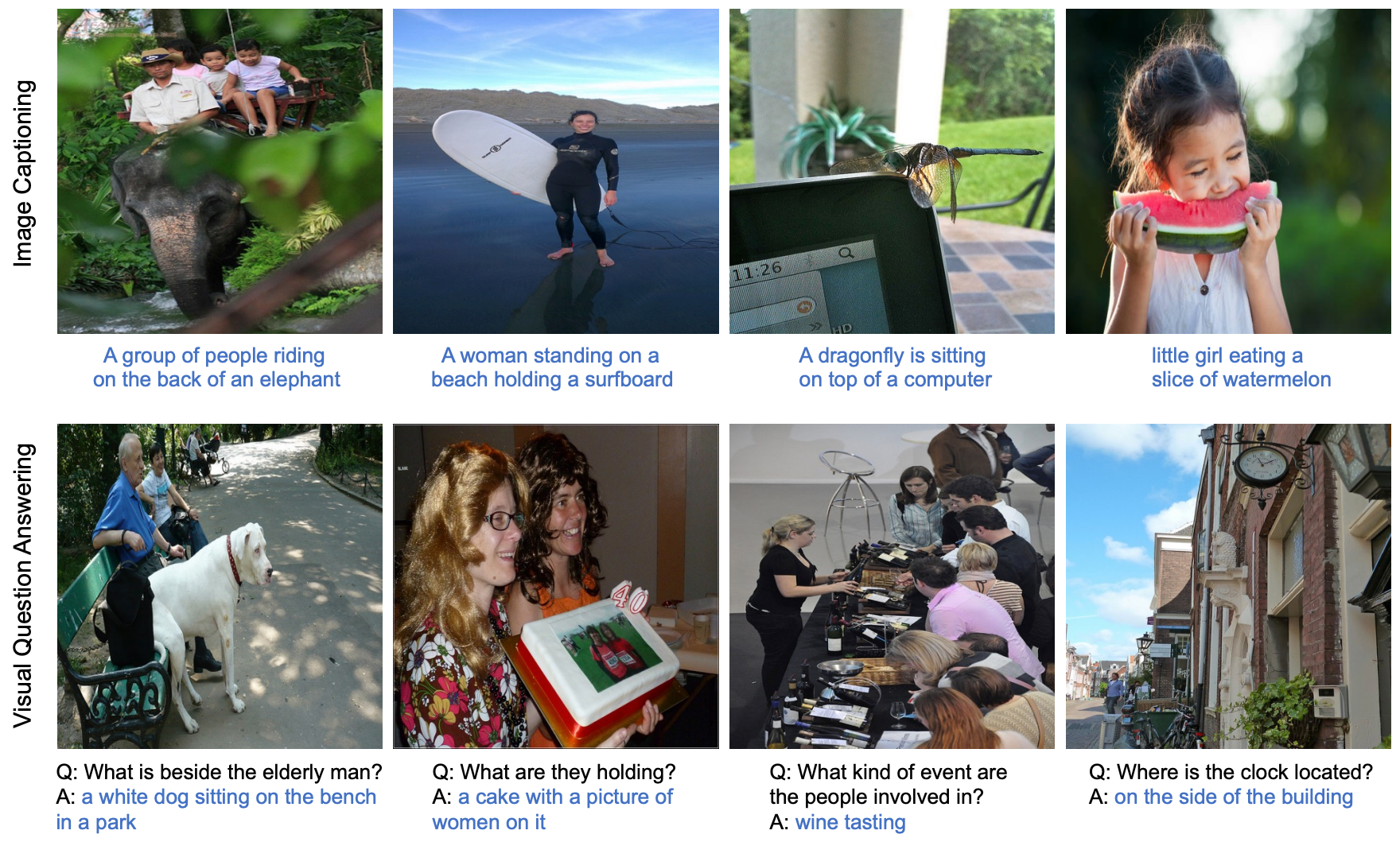
Qualitative evaluation: image generation
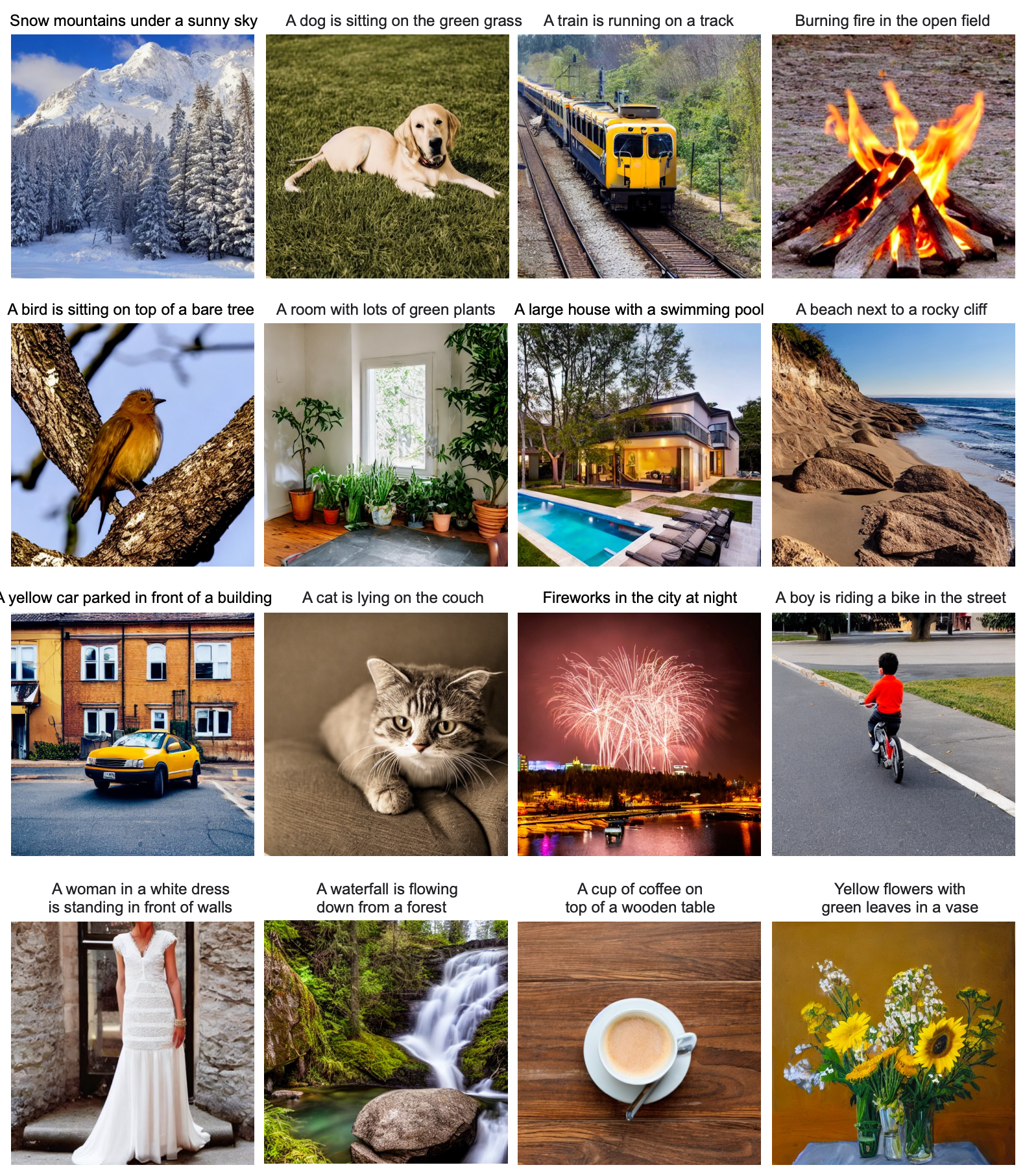
Citation
@article{ge2023planting, title={Planting a seed of vision in large language model}, author={Ge, Yuying and Ge, Yixiao and Zeng, Ziyun and Wang, Xintao and Shan, Ying}, journal={arXiv preprint arXiv:2307.08041}, year={2023} }
Get to know more about our Project SEED.
Acknowledgements
We sincerely acknowledge Sijie Zhao and Chen Li for their engaging discussions.
The website template was borrowed from Open X-Embodiment.